Integration of AI into Medical Diagnostics: A Revolution in Healthcare
Artificial Intelligence (AI) is reshaping the landscape of medical diagnostics, offering unprecedented accuracy, speed, and personalization in disease detection. From advanced imaging analysis to predictive analytics, AI is transforming the way healthcare professionals diagnose, treat, and manage diseases. Rather than replacing human expertise, AI augments it, improving diagnostic precision, enabling tailored treatments, and driving efficiency. As AI continues to evolve, its potential to revolutionize healthcare becomes even clearer—ushering in a future where medicine is predictive, preventative, and personalized. AI is no longer the future of healthcare; it is the present.
In this blog, we explore how AI is making a significant impact in healthcare diagnostics, from enhanced imaging analysis to predictive analytics for early disease detection. And will also explore the future possibilities of AI in healthcare.
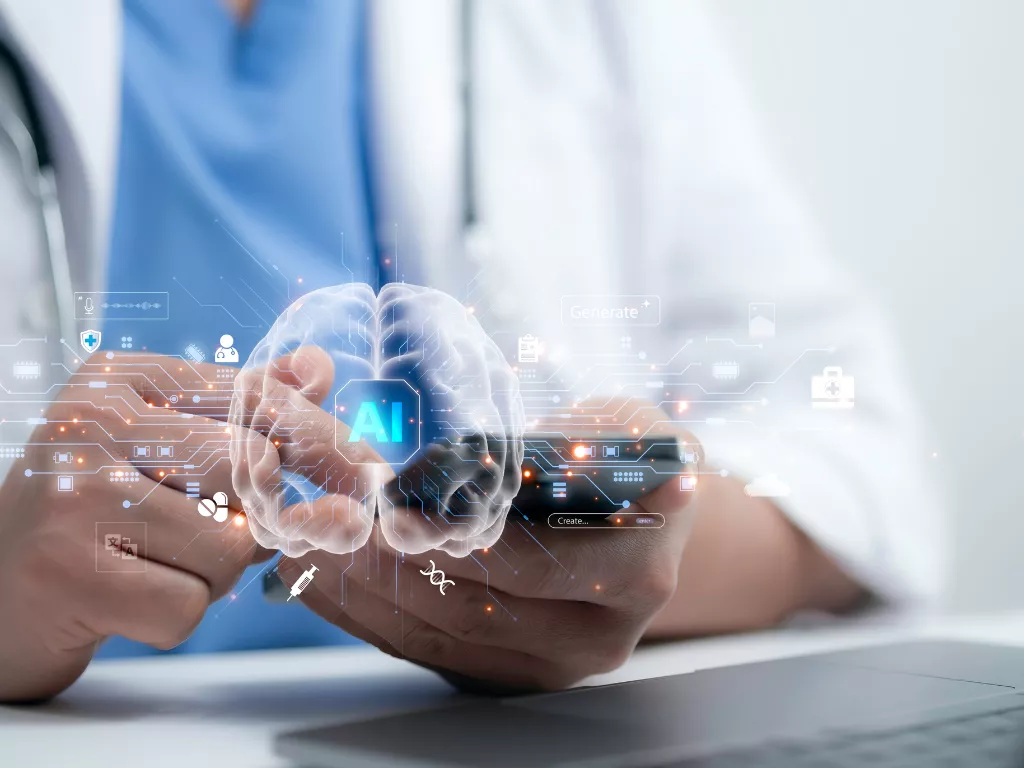
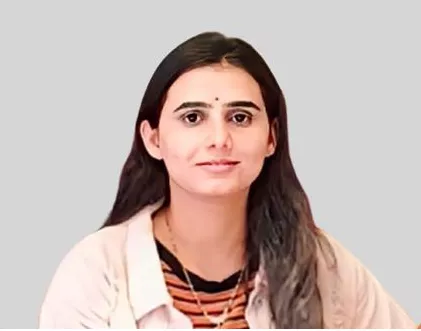
This blog is written by Laxmi Swami, Senior Software Engineer at Deocs. She specializes in debugging and enhancing various modules of the Otosuite and Noah applications. With expertise in WPF, MVVM, the Community Toolkit, and .NET, she brings extensive experience in developing desktop applications. Her strong analytical and problem-solving skills help her deliver reliable and efficient software solutions that meet client needs.
Decos is a cutting-edge technology services partner, addressing diverse industry needs across various, including medical domain. If you have any questions or would like advice on your project or proof of concept (POC), contact Devesh Agarwal. We'd love to connect with you!
Discover more
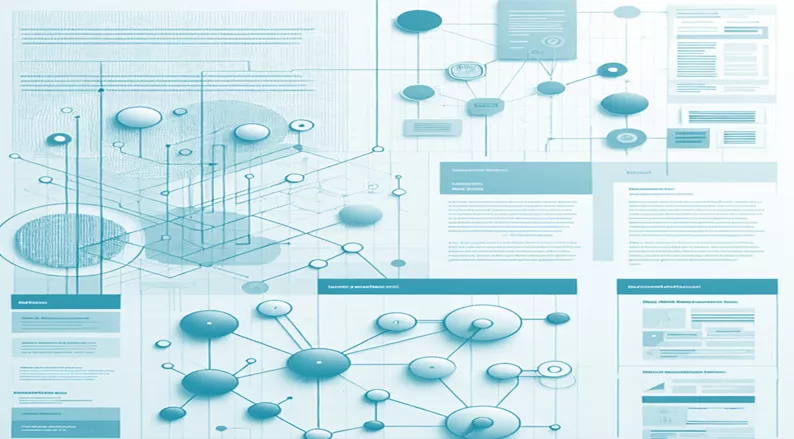
Building Better Digital Experiences with Information Architecture
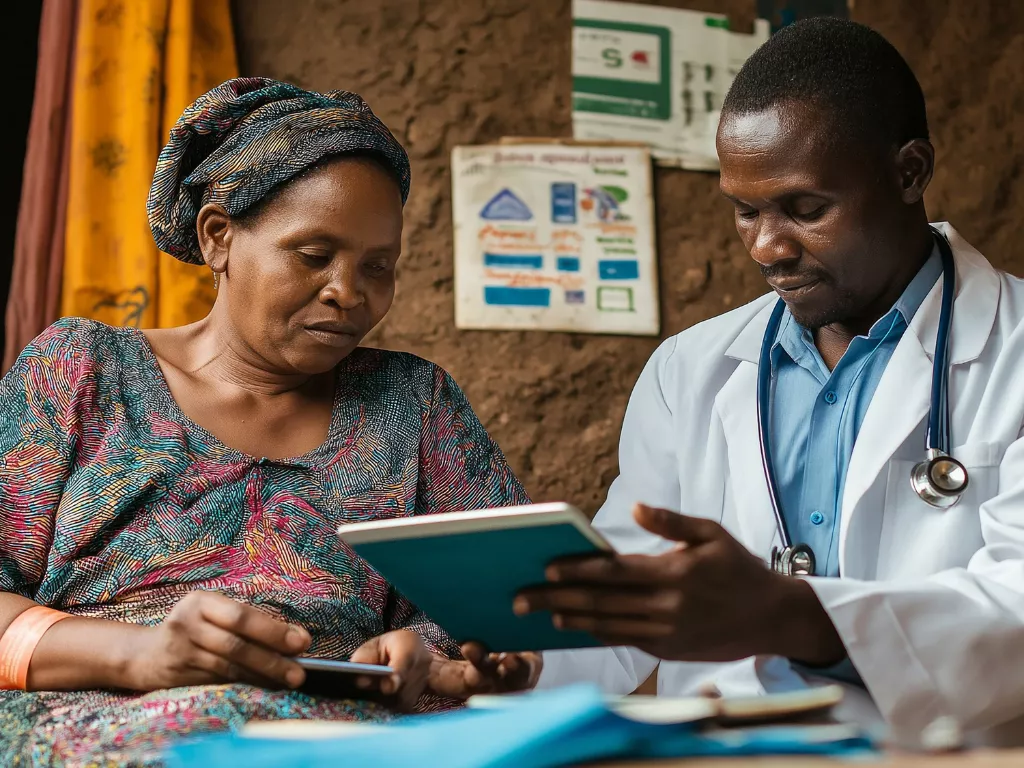
Revolutionizing Rural Healthcare: How AI is Shaping the Future of Telemedicine
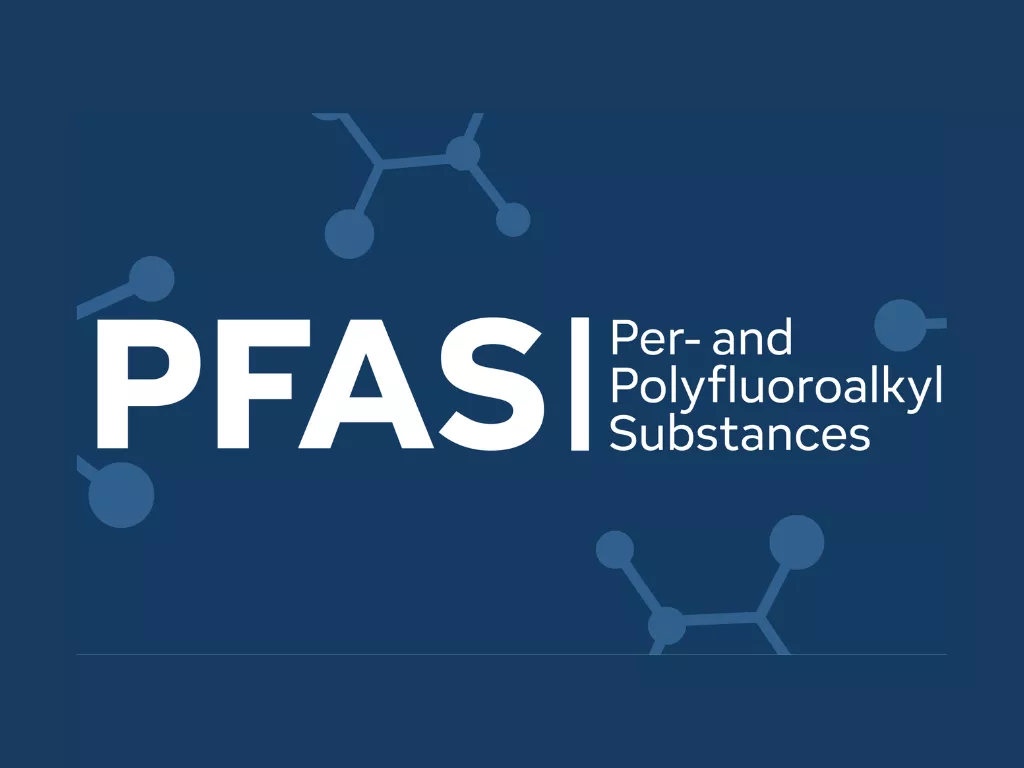